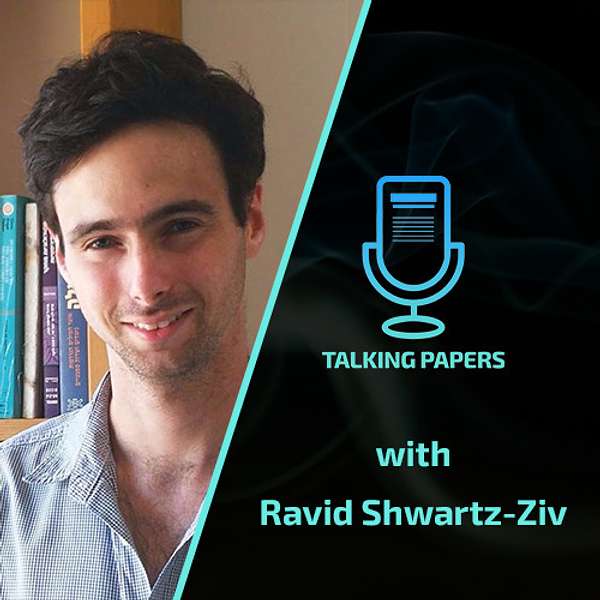
Talking Papers Podcast
🎙️ Welcome to the Talking Papers Podcast: Where Research Meets Conversation 🌟
Are you ready to explore the fascinating world of cutting-edge research in computer vision, machine learning, artificial intelligence, graphics, and beyond? Join us on this podcast by researchers, for researchers, as we venture into the heart of groundbreaking academic papers.
At Talking Papers, we've reimagined the way research is shared. In each episode, we engage in insightful discussions with the main authors of academic papers, offering you a unique opportunity to dive deep into the minds behind the innovation.
📚 Structure That Resembles a Paper 📝
Just like a well-structured research paper, each episode takes you on a journey through the academic landscape. We provide a concise TL;DR (abstract) to set the stage, followed by a thorough exploration of related work, approach, results, conclusions, and a peek into future work.
🔍 Peer Review Unveiled: "What Did Reviewer 2 Say?" 📢
But that's not all! We bring you an exclusive bonus section where authors candidly share their experiences in the peer review process. Discover the insights, challenges, and triumphs behind the scenes of academic publishing.
🚀 Join the Conversation 💬
Whether you're a seasoned researcher or an enthusiast eager to explore the frontiers of knowledge, Talking Papers Podcast is your gateway to in-depth, engaging discussions with the experts shaping the future of technology and science.
🎧 Tune In and Stay Informed 🌐
Don't miss out on the latest in research and innovation.
Subscribe and stay tuned for our enlightening episodes. Welcome to the future of research dissemination – welcome to Talking Papers Podcast!
Enjoy the journey! 🌠
#TalkingPapersPodcast #ResearchDissemination #AcademicInsights
Talking Papers Podcast
Reverse Engineering SSL - Ravid Shwartz-Ziv
Welcome to another exciting episode of the Talking Papers Podcast! In this episode, we delve into the fascinating world of self-supervised learning with our special guest, Ravid Shwartz-Ziv. Together, we explore and dissect their research paper titled "Reverse Engineering Self-Supervised Learning," published in NeurIPS 2023.
Self-supervised learning (SSL) has emerged as a game-changing technique in the field of machine learning. However, understanding the learned representations and their underlying mechanisms has remained a challenge - until now. Ravid Shwartz-Ziv's paper provides an in-depth empirical analysis of SSL-trained representations, encompassing various models, architectures, and hyperparameters.
The study uncovers a captivating aspect of the SSL training process - its inherent ability to facilitate the clustering of samples based on semantic labels. Surprisingly, this clustering is driven by the regularization term in the SSL objective. Not only does this process enhance downstream classification performance, but it also exhibits a remarkable power of data compression. The paper further establishes that SSL-trained representations align more closely with semantic classes than random classes, even across different hierarchical levels. What's more, this alignment strengthens during training and as we venture deeper into the network.
Join us as we discuss the insights gained from this exceptional research. One remarkable aspect of the paper is its departure from the trend of focusing solely on outperforming competitors. Instead, it dives deep into understanding the semantic clustering effect of SSL techniques, shedding light on the underlying capabilities of the tools we commonly use. It is truly a genre of research that holds immense value.
During our conversation, Ravid Shwartz-Ziv - a CDS Faculty Fellow at NYU Center for Data Science - shares their perspectives and insights, providing an enriching layer to our exploration. Interestingly, despite both of us being in Israel at the time of recording, we had never met in person, highlighting the interconnectedness and collaborative nature of the academic world.
Don't miss this thought-provoking episode that promises to expand your understanding of self-supervised learning and its impact on representation learning mechanisms. Subscribe to our channel now, join the discussion, and let us know your thoughts in the comments below!
All links and resources are available in the blogpost: https://www.itzikbs.com/revenge_ssl
🎧Subscribe on your favourite podcast app: https://talking.papers.podcast.itzikbs.com
📧Subscribe to our mailing list: http://eepurl.com/hRznqb
🐦Follow us on Twitter: https://twitter.com/talking_papers
🎥YouTube Channel: https://bit.ly/3eQOgwP