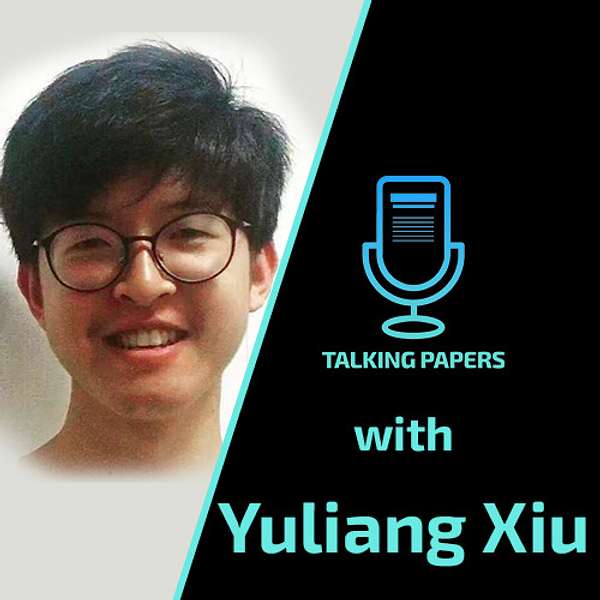
Talking Papers Podcast
🎙️ Welcome to the Talking Papers Podcast: Where Research Meets Conversation 🌟
Are you ready to explore the fascinating world of cutting-edge research in computer vision, machine learning, artificial intelligence, graphics, and beyond? Join us on this podcast by researchers, for researchers, as we venture into the heart of groundbreaking academic papers.
At Talking Papers, we've reimagined the way research is shared. In each episode, we engage in insightful discussions with the main authors of academic papers, offering you a unique opportunity to dive deep into the minds behind the innovation.
📚 Structure That Resembles a Paper 📝
Just like a well-structured research paper, each episode takes you on a journey through the academic landscape. We provide a concise TL;DR (abstract) to set the stage, followed by a thorough exploration of related work, approach, results, conclusions, and a peek into future work.
🔍 Peer Review Unveiled: "What Did Reviewer 2 Say?" 📢
But that's not all! We bring you an exclusive bonus section where authors candidly share their experiences in the peer review process. Discover the insights, challenges, and triumphs behind the scenes of academic publishing.
🚀 Join the Conversation 💬
Whether you're a seasoned researcher or an enthusiast eager to explore the frontiers of knowledge, Talking Papers Podcast is your gateway to in-depth, engaging discussions with the experts shaping the future of technology and science.
🎧 Tune In and Stay Informed 🌐
Don't miss out on the latest in research and innovation.
Subscribe and stay tuned for our enlightening episodes. Welcome to the future of research dissemination – welcome to Talking Papers Podcast!
Enjoy the journey! 🌠
#TalkingPapersPodcast #ResearchDissemination #AcademicInsights
Talking Papers Podcast
Yuliang Xiu - ICON
In this episode of the Talking Papers Podcast, I hosted Yuliang Xiu to chat about his paper "ICON: Implicit Clothed humans Obtained from Normals”, published in CVPR 2022. SMPL(-X) body model to infer clothed humans (conditioned on the normals). Additionally, they propose an inference-time feedback loop that alternates between refining the body's normals and the shape.
PAPER TITLE
"ICON: Implicit Clothed humans Obtained from Normals" https://bit.ly/3uXe6Yw
AUTHORS
Yuliang Xiu, Jinlong Yang, Dimitrios Tzionas, Michael J. Black
ABSTRACT
Current methods for learning realistic and animatable 3D clothed avatars need either posed 3D scans or 2D images with carefully controlled user poses. In contrast, our goal is to learn an avatar from only 2D images of people in unconstrained poses. Given a set of images, our method estimates a detailed 3D surface from each image and then combines these into an animatable avatar. Implicit functions are well suited to the first task, as they can capture details like hair and clothes. Current methods, however, are not robust to varied human poses and often produce 3D surfaces with broken or disembodied limbs, missing details, or non-human shapes. The problem is that these methods use global feature encoders that are sensitive to global pose. To address this, we propose ICON ("Implicit Clothed humans Obtained from Normals"), which, instead, uses local features. ICON has two main modules, both of which exploit the SMPL(-X) body model. First, ICON infers detailed clothed-human normals (front/back) conditioned on the SMPL(-X) normals. Second, a visibility-aware implicit surface regressor produces an iso-surface of a human occupancy field. Importantly, at inference time, a feedback loop alternates between refining the SMPL(-X) mesh using the inferred clothed normals and then refining the normals. Given multiple reconstructed frames of a subject in varied poses, we use SCANimate to produce an animatable avatar from them. Evaluation on the AGORA and CAPE datasets shows that ICON outperforms the state of the art in reconstruction, even with heavily limited training data. Additionally, it is much more robust to out-of-distribution samples, e.g., in-the-wild poses/images and out-of-frame cropping. ICON takes a step towards robust 3D clothed human reconstruction from in-the-wild images. This enables creating avatars directly from video with personalized and natural pose-dependent cloth deformation.
RELATED PAPERS
📚 Monocular Real-Time Volumetric Performance Capture https://bit.ly/3L2S4JF
📚 PIFu https://bit.ly/3rBsrYN
📚 PIFuHD https://bit.ly/3rymDiE
LINKS AND RESOURCES
💻 Project Page https://icon.is.tue.mpg.de/
💻 Code https://github.com/yuliangxiu/ICON
To stay up to date with Yulian'gs latest research, follow him on:
👨🏻🎓 Yuliang's personal page: https://bit.ly/3jQb16n
🎓 Google Scholar: https://bit.ly/3JW25ae
🐦 Twitter: https://twitter.com/yuliangxiu
👨🏻🎓LinkedIn: https://www.linkedin.com/in/yuliangxiu/
Recorded on March11th 2022.
CONTACT
If you would like to be a guest, sponsor or just share your thoughts, feel free to reach out via email: talking.papers.podcast@gmail.com
SUBSCRIBE AND FOLLOW
🎧Subscribe on your favourite podcast app: https://talking.papers.podcast.itzikb...
📧Subscribe to our mailing list: http://eepurl.com/hRznqb
🐦Follow us on Twitter: https://twitter.com/talking_papers
🎧Subscribe on your favourite podcast app: https://talking.papers.podcast.itzikbs.com
📧Subscribe to our mailing list: http://eepurl.com/hRznqb
🐦Follow us on Twitter: https://twitter.com/talking_papers
🎥YouTube Channel: https://bit.ly/3eQOgwP