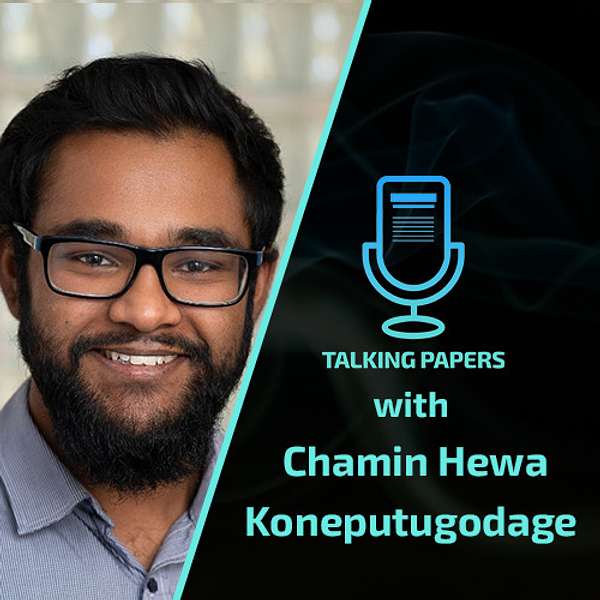
Talking Papers Podcast
🎙️ Welcome to the Talking Papers Podcast: Where Research Meets Conversation 🌟
Are you ready to explore the fascinating world of cutting-edge research in computer vision, machine learning, artificial intelligence, graphics, and beyond? Join us on this podcast by researchers, for researchers, as we venture into the heart of groundbreaking academic papers.
At Talking Papers, we've reimagined the way research is shared. In each episode, we engage in insightful discussions with the main authors of academic papers, offering you a unique opportunity to dive deep into the minds behind the innovation.
📚 Structure That Resembles a Paper 📝
Just like a well-structured research paper, each episode takes you on a journey through the academic landscape. We provide a concise TL;DR (abstract) to set the stage, followed by a thorough exploration of related work, approach, results, conclusions, and a peek into future work.
🔍 Peer Review Unveiled: "What Did Reviewer 2 Say?" 📢
But that's not all! We bring you an exclusive bonus section where authors candidly share their experiences in the peer review process. Discover the insights, challenges, and triumphs behind the scenes of academic publishing.
🚀 Join the Conversation 💬
Whether you're a seasoned researcher or an enthusiast eager to explore the frontiers of knowledge, Talking Papers Podcast is your gateway to in-depth, engaging discussions with the experts shaping the future of technology and science.
🎧 Tune In and Stay Informed 🌐
Don't miss out on the latest in research and innovation.
Subscribe and stay tuned for our enlightening episodes. Welcome to the future of research dissemination – welcome to Talking Papers Podcast!
Enjoy the journey! 🌠
#TalkingPapersPodcast #ResearchDissemination #AcademicInsights
Talking Papers Podcast
DiGS - Chamin Hewa Koneputugodage
In this episode of the Talking Papers Podcast, I hosted Chamin Hewa Koneputugodage to chat about OUR paper "DiGS: Divergence guided shape implicit neural representation for unoriented point clouds”, published in CVPR 2022.
In this paper, we took on the task of surface reconstruction using a novel divergence-guided approach. Unlike previous methods, we do not use normal vectors for supervision. To compensate for that, we add a divergence minimization loss as a regularize to get a coarse shape and then anneal it as training progresses to get finer detail. Additionally, we propose two new geometric initialization for SIREN-based networks that enable learning shape spaces.
PAPER TITLE
"DiGS: Divergence guided shape implicit neural representation for unoriented point clouds"
AUTHORS
Yizhak Ben-Shabat, Chamin Hewa Koneputugodage, Stephen Gould
ABSTRACT
Shape implicit neural representations (INR) have recently shown to be effective in shape analysis and reconstruction tasks. Existing INRs require point coordinates to learn the implicit level sets of the shape. When a normal vector is available for each point, a higher fidelity representation can be learned, however normal vectors are often not provided as raw data. Furthermore, the method's initialization has been shown to play a crucial role for surface reconstruction. In this paper, we propose a divergence guided shape representation learning approach that does not require normal vectors as input. We show that incorporating a soft constraint on the divergence of the distance function favours smooth solutions that reliably orients gradients to match the unknown normal at each point, in some cases even better than approaches that use ground truth normal vectors directly. Additionally, we introduce a novel geometric initialization method for sinusoidal INRs that further improves convergence to the desired solution. We evaluate the effectiveness of our approach on the task of surface reconstruction and shape space learning and show SOTA performance compared to other unoriented methods.
RELATED PAPERS
📚 DeepSDF
📚 SIREN
LINKS AND RESOURCES
💻 Project Page
💻 Code
🎥 5 min video
To stay up to date with Chamin's latest research, follow him on:
🐦 Twitter
👨🏻🎓LinkedIn
Recorded on April 1st 2022.
CONTACT
If you would like to be a guest, sponsor or just share your thoughts, feel free to reach out via email: talking.papers.podcast@gmail.com
SUBSCRIBE AND FOLLOW
🎧Subscribe on your favourite podcast app
📧Subscribe to our mailing list
🐦Follow us on Twitter
🎥YouTube Channel
#talkingpapers #CVPR2022 #DiGS #NeuralImplicitRepresentation #SurfaceReconstruction #ShapeSpace #3DVision #ComputerVision #AI #DeepLearning #MachineLearning #deeplearning #A
🎧Subscribe on your favourite podcast app: https://talking.papers.podcast.itzikbs.com
📧Subscribe to our mailing list: http://eepurl.com/hRznqb
🐦Follow us on Twitter: https://twitter.com/talking_papers
🎥YouTube Channel: https://bit.ly/3eQOgwP